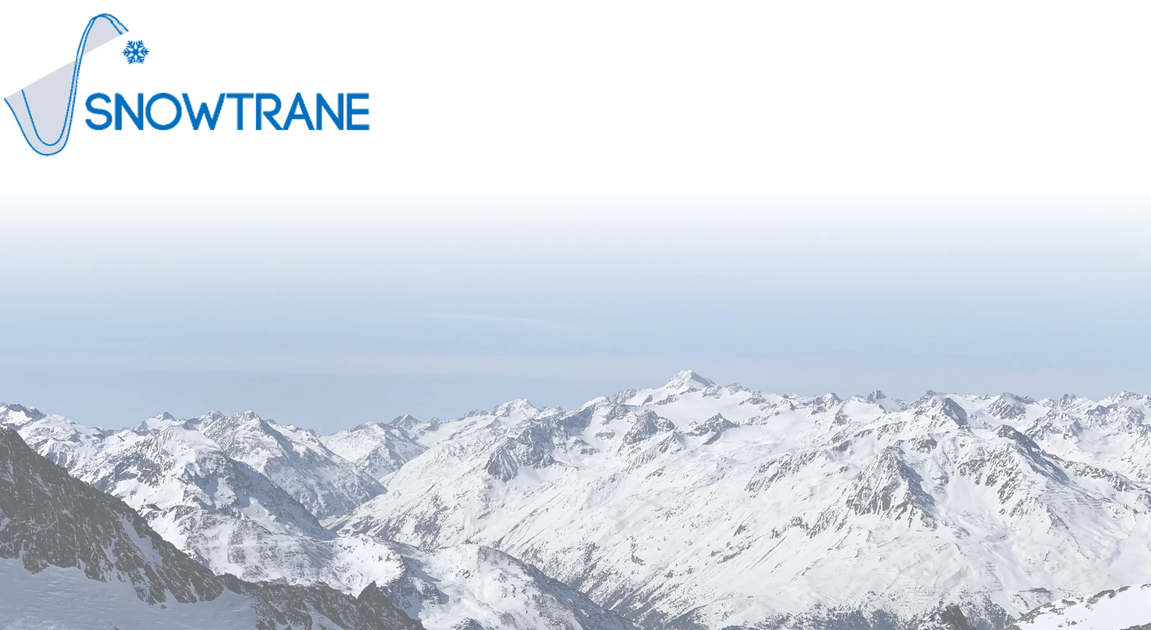
Snowtrane
Snowtrane
Snow mass retrieval by training machine learning algorithms with Earth Observation data
Our mission
Knowledge about the amount of water stored in seasonal snow is critical to asses global water resources. More than 1 billion people worldwide depend on drinking water from seasonal snow. Additonally, this water fuels hydropower generation, supports agriculture and industry, but it also causes hazards to mountain communities such as avalanches or floods. Finally, snow plays an indispensable role in regulating Earths' energy budget.
With our project, we want to contribute to a better understanding of how much snow is seasonally stored in mountainous snowpacks. We strive to deliver unprecedented, high-resolution snow products by combining ESA Sentinel-1 satellite data with other explainable variables in a Machine Learning approach. Our focus will be on two key snow products, namely Snow Depth (SD) and the Snow Water Equivalent (SWE).
Objectives
Our project will focus on 2 key snow products of value to stakeholders and industry. During this process, we want to gain insight in the relationship between microwave-based satellite data and snow.
High-resolution Snow Depth estimates
The first objective of Snowtrane is to improve upon the current state-of-the-art snow depth estimates over vast mountainous areas, by combining Sentinel-1 data with other explainable variables in a Machine Learning setup.
High-resolution SWE estimates
In our project, we aim to make direct estimates of the snow water equivalent (SWE), the variable of most critical need for informed decision making. Hereby, we argue that a Machine Learning approach that uses input variables related to snow density, needed to convert Snow Depth to SWE, is capable of directly providing accurate SWE estimates over mountainous areas.
Improved understanding of the satellite observations/snow relationship
Analysis of machine learning outputs will help us better understand when and where satellite observations are used to correlate with snow, to understand the snow satellite interaction.
Methods
1Collecting training and validation data
An extensive dataset of SD and SWE measurements, along with corresponding input variables is collected and cleaned to train and validate our machine learning models.
2Selecting and training appropriate machine learning models
An appropriate model is selected following a model comparison phase, after which the model is trained with the collected dataset.
3Validating with snow measurements
The perfomance of the selected model is verified by spatial and temporal cross validation and by comparing spatial outputs with aeroplane photogrammetry images.
4Producing and distributing snow datasets
Snow estimates are produced for the selected mountain ranges spanning multiple years.
Team
Our project is supported by team members of both Ghent University and the Catholic University of Leuven. Please contact us for further information!
PhD researcher | UGent, Hydro-Climate Extremes lab and KULeuven, Department of Earth and Environmental Sciences
My function in the project is to collect input variables for training the Machine Learning models, produce spatial and temporal SD and SWE estimates, and validate the outcomes with measurements taken on site in the mountain ranges. Next, I am responsible for processing Sentinel-1 polarimetric variables and collecting snow measurements from various mountain ranges and providers.
Postdoc | KULeuven, Department of Earth and Environmental Sciences
Currently, I am a postdoctoral researcher at KU Leuven. During my PhD I used a variety of tools including climate and snow models, field and satellite observations, and Machine Learning to explore water on Earth's ice sheets. I am currently working on a project using synthetic aperture radar satellite imagery, Machine Learning, and data assimilation to improve estimates of snow depth and stream flow in the European Alps.
Postdoc | UGent, Hydro-Climate Extremes lab
As the coordinator of the Snowtrane project, my main task is to support the team members with their research on developing remote sensing retrieval algorithms for snow. Furthermore, I am responsible for the communication of project results to BELSPO and the snow remote sensing community.
Professor| KULeuven, Department of Earth and Environmental Sciences
Our group’s objective is to estimate land surface variables, including snow, using remote sensing, modeling and data assimilation. My role in Snowtrane is to support Lucas and Devon with their PhD and postdoctoral research, and educate the new generation scientists on the importance of snow and the methods to estimate it (modeling, earth observation, field campaigns).
Publications
Dunmire, D., Lievens, H., Boeykens, L., and De Lannoy, G. 2024. A Machine Learning Approach for Estimating Snow Depth Across the European Alps from Sentinel-1 Imagery. Remote Sensing of Environment , 314(2024):114369. https://doi.org/10.1016/j.rse.2024.114369
About us
Snowtrane is a project funded by the Belgian Science Policy Office (BELSPO) and aims to produce unprecedented high-resolution snow products.
Contact
UGent: Department of Environment
Campus Coupure - Block A
Coupure links 653, B-9000 Gent
KULeuven: Department of Earth and Environmental Science
Campus Arenberg - GEO-Instituut
Celestijnenlaan 200E, B-3001 Leuven-Heverlee
© 2024